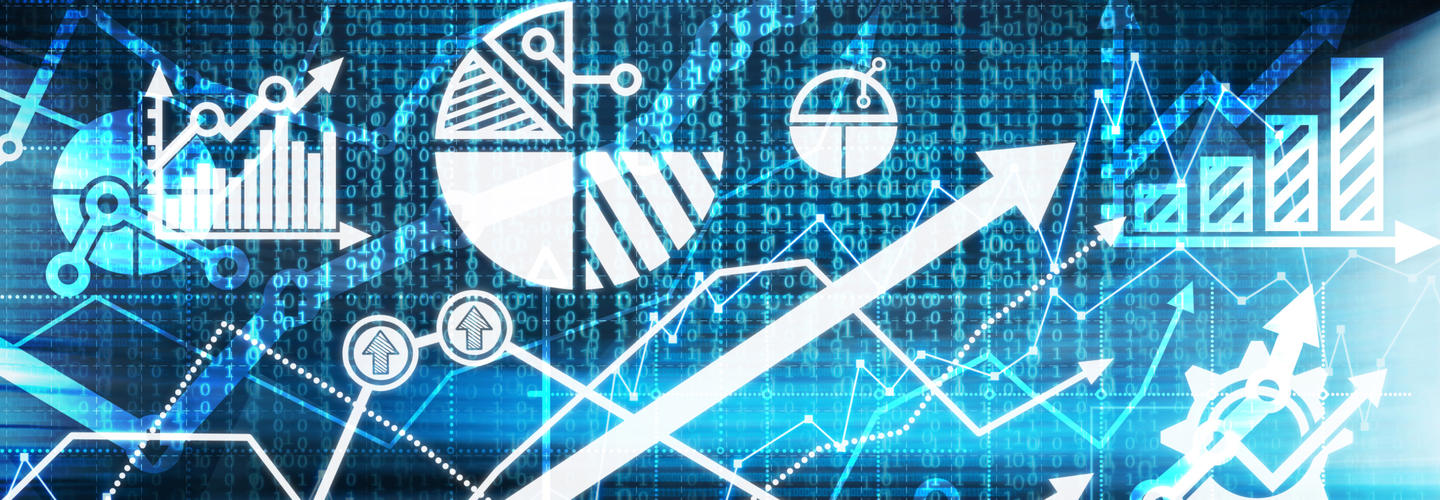
Analytics in Banking Industry
The level of analytical maturity of many financial institutions is very low. They have to make quick advancements, because if they do not take the necessary actions the fintech startups or their main competitors will take more space up in the market.
Since 2010, there have been conversations about the analytical maturity of companies, taking data and information to take action. The level of maturity is associated with how much participation of automated processes there is, intervention of people and decision-making, all before the specific action.
In 2013 Gartner presented a diagram that graphically described the concept:
The diagram shows 4 levels:
- Descriptive: It allows the company to answer what happened by using standard reports, ad-hoc reports and visualizations only.
- Diagnostic: It allows the company to answer why did it happen. The company uses OLAP tools, or Self-service BI and some kind of analysis.
- Predictive: It allows the company to answer what will happen. In this case, they use predictive models, what-if and forecast tools, and simulations can be done.
- Prescriptive: in this level the organization will know what they should do by using optimization models and heuristics.
But, in 2017 with the advances in the Artificial intelligence a new level is added: the cognitive level.
With the cognitive level the company will know which the best action to take is by using natural language, Machine Learning, Deep Learning or Cognitive Computing.
Taking this maturity model, analyzing the data that arises from multiple reports on the financial sector, we see that they continue to be at a very low level of automation and in the use of analytical tools.
In the report What’s Going on in Banking 2021 by Cornerstone Advisors we can still see that 52% of the Banks and 35% of the Credit Unions have not launched a digital transformation strategy and of this, only 5% have fully completed one. Only 6% have developed processes at the cognitive level.
As Ron Shelvin says in his Forbes column, “With heavy reliance on vendors for their technology applications and relatively small IT shops (compared to the top 25 banks, that it), most mid-size banks and credit unions don’t have the internal resources to experiment with and develop AI-based solutions.” We can see that the incorporation of cognitive technologies in these institutions has not advanced as they need to, and if they do not, they will end up being displaced by their large competitors.
In my experience, this happens because all maturity levels are not transited, and it is intended to follow a trend and incorporate the technology of the moment without analyzing the real value that it incorporates. It is very common for a senior manager to hear that other colleagues are doing Artificial Intelligence projects and go back to his company and tell, or almost force, their collaborators to implement something, only with the desire to later be able to be in another meeting and say his organization already has it.
These Banks and Credit Unions could be wondering if transiting these levels involves years of implementations. The answer to that question is no. With agile methodologies, industry use cases, minimal viable products, analytical methodologies that allow evolutionary systems, these levels can quickly be transited. They can be incorporated in areas where the impact is greater. The lessons learned internally and externally are used, this means that what worked for one Credit Union does not have to work for another, but they can learn from that case. With the right strategy the analytical technologies that respond to market needs could be incorporated quickly. If I want to have a significant impact in Loan Productivity, Overall Customer Retention, Loan Volume, Up-Sell/Cross-sell effectiveness, among other business metrics, I have to speed up my analytics-based decision making.
What is most important to achieve success is to work with a company that has the experience of working at all levels of analytical maturity, that has in-depth knowledge of the financial industry, its best practices, success stories, but at the same time, count with use cases from other industries that allow it to “cross-pollinate” in terms of innovation and thus have constant innovations.
Leonardo Loureiro
CEO at Quanam USA