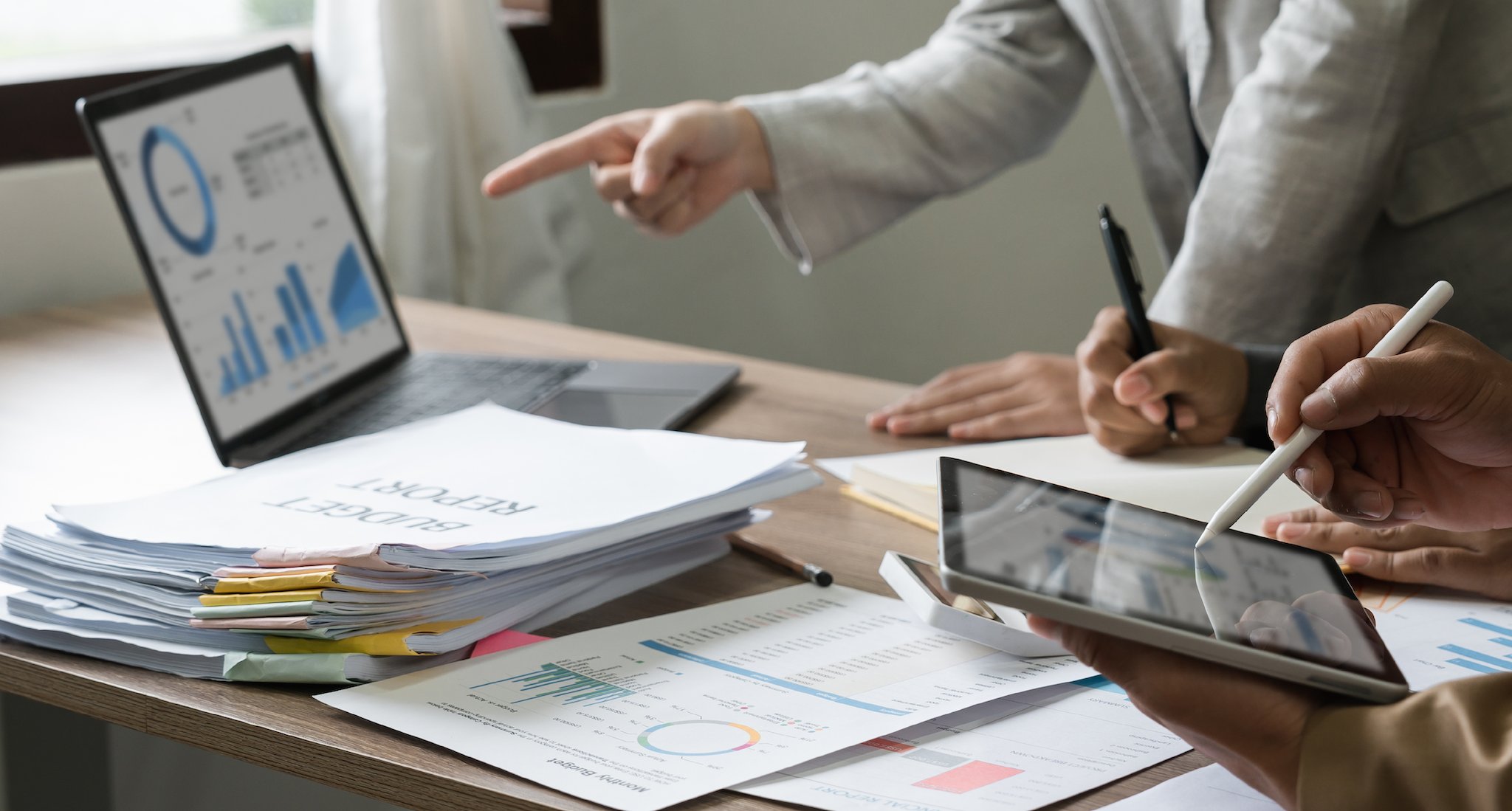
Navigating Data Governance: strategies for every stage in the journey
Organizations often mistakenly believe implementing a Data Governance program involves embarking on large-scale, high-risk projects. In this context, it is crucial to start with quick wins. Advancing with quick wins allows us to improve incrementally, prioritizing according to business objectives and reducing resistance to change. For this, it is necessary to define a business case aligned with the organization’s strategy to demonstrate that through data governance we can help monetize the use of data.
In which organization are there no business cases that have difficulties related to data quality? It is common for metadata and data quality management to be among the first to be executed.
Metadata Management
Before discussing management, we must define what we mean by metadata. The technical definition tells us that they are “data about data”. The goal of metadata management is to turn that data into information and knowledge about the data that an organization has. There are different types of metadata that we can classify into two groups.
- The Data Dictionaries state the Technical Metadata. They provide details about source and destination systems, database table and field structures, and dependencies of various types of assets, such as host systems, ETL processes, databases, and data files, among others.
- The Business Glossary states the Business Metadata, which includes terms, information governance rules, and labels that provide a context of information that allows effective communication within the organization.
- The Data Catalogue is a bridge between Technical and Business Metadata, functioning as a proactive tool. It enables users to engage in various actions, including data profiling, permission requests, search execution, and the creation of community feedback. Its purpose is to link the business terminology and its relationship with the data in the systems.
More user-friendly than a Data Dictionary, the Data Catalogue offers both business and technical users the ability to access it, encouraging self-service.
Data Quality Management
Identifying data quality problems and defining appropriate actions to improve them cannot be an effort of a group of people isolated within the organization, but corporate. Its goal is to achieve and maintain high levels of data quality in the critical data of the organization. To detect quality problems, there are two possible approaches:
From the Business
This approach looks for poor-quality data problems that negatively impact the organization’s business processes. Examples of this approach involve complaints, customer loss, incorrect decisions, and lack of customer contact data. A disadvantage of this approach is that we must be very close to the business to detect these problems and requires effort and time to analyze if the causes are due to data problems.
From the Data
This method involves identifying data issues by establishing a set of quality criteria the data must fulfill. Understanding the data’s definition, potential values, and relationships is critical. The subsequent phase involves conducting data profiling to either verify the created metadata or to supplement it. When the data’s quality is ascertained, business analysts apply these criteria to uncover evidence of data issues. This crucial information aids in probing and rectifying the underlying causes of these issues. Compared to the previous method, this one is more feasible as it demands less time, involves a broader group in problem detection, and can identify issues that might go unnoticed by the other method. However, it’s important to note that data may comply with these rules yet still be unsuitable for business processes, leading to negative impacts before the issue is fully recognized.
The data approach is inherently proactive, emphasizing the synergy between defining catalogue terms and establishing compliance rules. This strategy prioritizes data profiling and monitoring as initial steps, leading to identifying and correcting issues. A robust data quality program should integrate both this and the business-centric approach to ensure the most comprehensive identification of existing data issues.
Conclusion
Both metadata management and data quality management are key activities within a Data Governance initiative. With proper management of both, we can define business terms, how they relate to systems, tables, reports, data models, and the associated quality rules to determine their level of confidence or potential problems that allow us to improve, prevent, and monitor data quality.
No matter the level of maturity of your organization in terms of Data Governance, you can always start walking the path through quick wins.
Gustavo Mesa
Engineer
Certified Data Management Professional (CDMP) by DAMA
Data Governance Practice Leader at Quanam