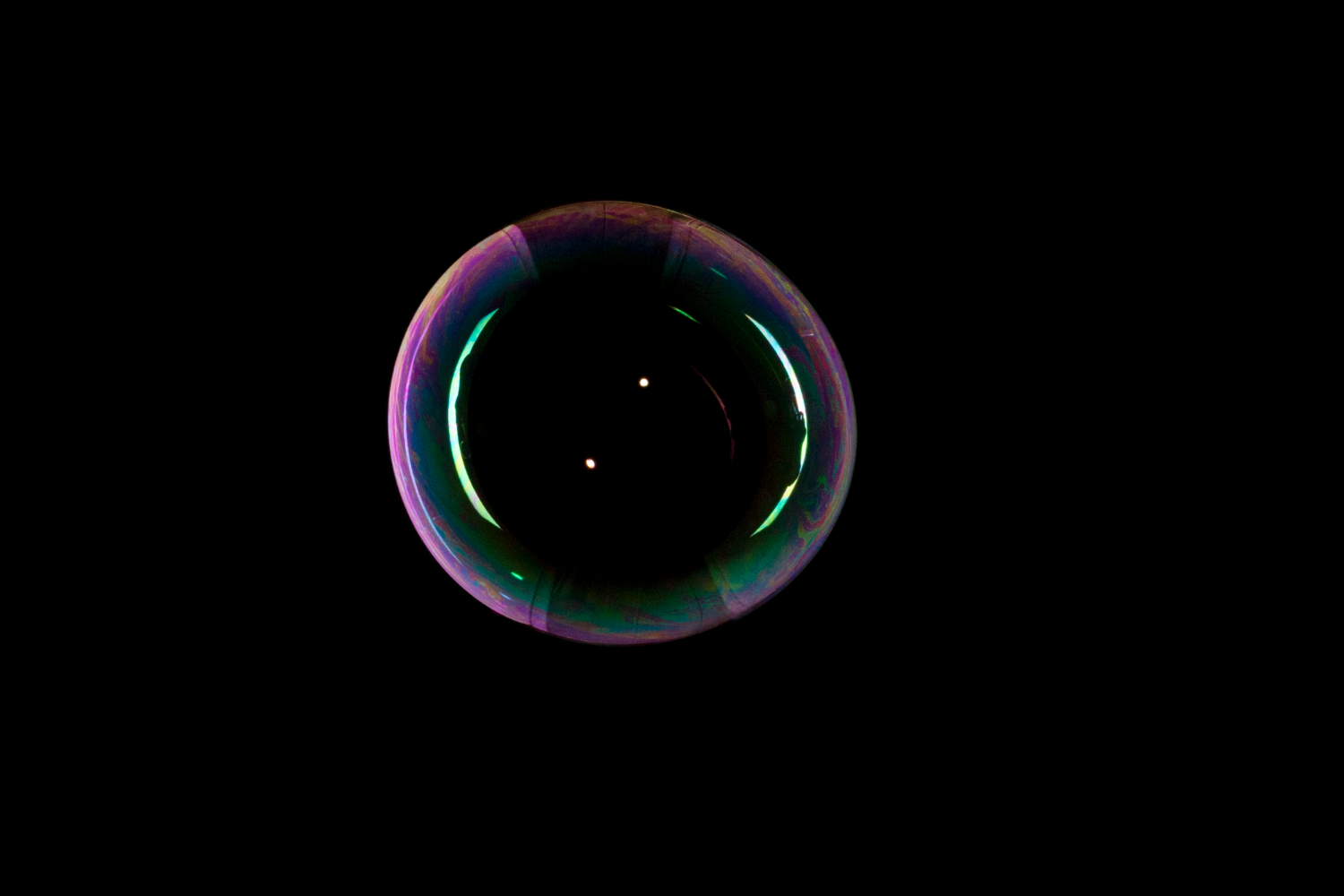
Is an AI bubble coming?
A few days ago, comments began to be heard warning about the market’s exaggerated optimism in relation to artificial intelligence, especially due to the huge investments in data center infrastructure.
Below I will reproduce two articles published less than ten days ago, the first one quoting Roger McNamee, a renowned American businessman and investor with global projection, and the second one with the considerations of Goldman Sachs’ strategists, both addressing this topic. Then I will make some considerations of my own about the expectations with artificial intelligence and the unquestionable opportunities that it creates in all areas of the economy and society.
Roger McNamee: is AI a fraud?
In a recent CNBC interview, on 07/08/2024, Roger McNamee, CEO of the venture capital firm Elevation Partners and an experienced technology investor, said that CAPEX in AI is being carried out based on projections that are difficult to evaluate and with great chances of not being confirmed. “The United States loves financial crazes,” he said. Elevation Partners was one of the first firms to invest money in Facebook. McNamee began his career at T. Row Price, where he led the science and technology fund. He was also a founder of Silver Lake Partners, the first private equity fund focused on technology companies, before founding Elevation Partners.
For McNamee, there is evidence of a bubble in artificial intelligence, and, if that is the case, sooner or later there will be an adjustment. “We are making assumptions about what that technology can do, but we can’t prove it, and we are applying it in places where it may not work,” McNamee said. “If this is the case, this craze will come to an end, and the biggest stocks in the S&P 500 will be hit hard.”
McNamee published his arguments in X, mentioning that investments in data centers already total US$ 100 billion so far and are growing at the rate of US$ 10 billion per month. “The biggest investors – Microsoft, NVIDIA, Google, Amazon, Meta – have tons of cash on hand and dominate the S&P 500. If they were wrong, it would be a disaster for the markets.”. McNamee highlighted that Goldman Sachs published a report “a few days ago” saying that investments in CAPEX are excessive. The document has a suggestive title: “GenAI: Much expense for a small benefit?”
“Goldman is not the first Wall Street firm to show concern. Barclays already did this, and Sequoia also published a report on CAPEX in AI, saying that the industry needs US$600 billion in revenue to justify the investments made in computing and cloud,” McNamee wrote. For him, “When companies like Sequoia and Goldman Sachs tell me that there is a bubble, I pay attention.”
But in his assessment, the reasons for distrusting the potential of AI are not limited to frustration with CAPEX expenses. McNamee cites the intense use of energy and water in producing and operating processors and in training language models. According to him, “A report suggests that half a liter of water is wasted every time you ask a chatbot a question.” For McNamee “GenAI is accelerating climate change. Microsoft and Google promised to be carbon neutral by 2030, [but] Microsoft’s carbon footprint has already increased 30% in two years, and Google’s, 45% in five years.”.
McNamee also cites aspects related to copyright, privacy, data security and misinformation. “Because of the GenAI craze, people are trying to apply large-scale language models (LLMs) on everything, including a wide range of cases where they will certainly fail,” McNamee said. “People will be harmed, and some will not recover,” he warned, in relation with fake news and deep fakes. “Safety should be a priority.”
For McNamee, AI models are not intelligent, they just “use statistics to guess the next most convenient word, paragraph or image. They only know what their training leaves them. What they do best is “bullshit”. There is more evidence that LLMs are a scam, and not the next big thing. Careful.“
As big techs dominate the S&P 500, McNamee concludes by stating that, when the AI bubble bursts, “Big Techs won’t go bankrupt, but all forecasts will collapse, dragging stocks down with them.”. “There is no way to know when the bubble will burst, but it will happen,” he said. “Be prepared.”
Goldman Sachs: there is no bubble (so far)
With tech stock prices soaring, Wall Street analysts are riddled daily with the same question: Is there an AI stock bubble after all? A team of Goldman Sachs’ strategists crunched the numbers and compared the current metrics of technology companies with those seen in the years of euphoria that preceded the Internet bubble. The conclusion, published three days later, contradicts McNamee’s controversial article: despite the large increase in capital and R&D expenditures, they are still “well below Internet bubble levels,” considering the cash flow of the companies.
According to Goldman, investors have expressed concern about possible “overinvestment” by so-called hyper-scalers, the big tech companies that are expanding the use of AI. These are Microsoft, Amazon, Google and Meta, which allocated $357 billion in capital expenditures and R&D in the last four quarters.
Hyper-scalers are ‘phase 2’ companies in the AI industry, according to Goldman’s classification. NVIDIA is considered ‘phase 1’. Big Techs are leading the stock market rally, with a 26% appreciation just this year, which explains investors’ apprehension.
“Hyper-scalers represent 23% of the total investments made by S&P 500 companies,” the analysts say. “These companies have signaled that they will allocate additional capital to achieve their AI goals.“
Could this be a warning sign? Not yet, according to Goldman’s analysts.
“Considering the profits of these companies, the cycle of capital spending on AI appears much smaller compared to the cycle realized in the Internet bubble,” the report says.
During the euphoria of the new economy, technology, media and telecommunications companies invested more than 100% of their cash flow, and they were companies that often did not even make a profit. “Today, by contrast, most large technology companies are extremely profitable,” the analysts say.
CAPEX currently represents 72% of these companies’ cash flow, and it has even fallen in margin, because profits have increased. According to Goldman, Big Techs will be able to maintain the recent average ROI if future earnings remain within projections.
Over the past five years, the average return on investment has been 33%. In comparison, the average return on investment for telecommunications between 1996 and 2000 was 19%.
“But as demonstrated in the tech bubble, sales revisions will be a key indicator for investors to assess the durability of AI trading,” the strategists wrote.
According to Goldman, many Internet and telecommunications companies saw their revenues collapse in the late 1990s. Others, such as Microsoft, continued to report higher profits. “Hyper-scalers will have to demonstrate that their investments will generate income and profits,” Goldman concludes. “Indications that these revenues and profits are not being generated, such as a downward revision to sales, could lead to a rating downgrade.“
Conclusions: where is the compass?
We already know that specialized media, market analysts and mainstream media tend to exaggerate and to amplify the possible effects on the economy of circumstances and milestones linked to technology. We all remember the apocalyptic vision of Y2K, when collapses of all kinds were anticipated due to the change of the first digit of the year from 12/31/1999 to 01/01/2000, and we know that, although Y2K was the source of enormous income for IT solution providers, in the end “the mountain gave birth to a mouse” and none of the predicted disasters occurred, not necessarily because everyone had adapted their systems.
More recently we experienced phenomena such as migration to the cloud, with opinion generators fueling the fire to convince Tyrians and Trojans that whoever did not migrate to the cloud would lose flexibility and competitiveness to such an extent that doing so was a matter of survival. This migration, of which few said that one of its objectives was to increase the “wallet share” of clients in favor of hyper-scalers, big techs, business software manufacturers and data center owners, along with agility and flexibility also promised economies, which was not confirmed in practice, generating a wave of “rebound” meaning that numerous companies and public institutions around the world are considering returning to their own data centers.
Part of this saga was also the borderline irrational euphoria with startup or .com entrepreneurs that caused the internet bubble, which is now being compared to an alleged artificial intelligence bubble. In addition to the clear differences in relation to the financial situation, cash flow and profitability of the protagonists of the internet crisis, compared to the big techs that lead the explosion of artificial intelligence, we must consider that the acceleration of innovation’s pace has created several sources of proliferation of versions that end up creating some confusion about AI/GenAI. Let’s look at some examples.
- Foundational Models & LLMs: A Foundational Model and an LLM (Large Language Model) are closely related, but they are not the same thing, although they are often used interchangeably in some contexts. The distinction between the two can be subtle and depends on how each term is defined and used.
An LLM specifically refers to large-scale language models that are trained with huge data sets and have a large number of parameters. These models are designed to handle tasks related to natural language processing (NLP), such as text comprehension, text generation, translation, and other applications. Well-known examples of LLMs are GPT-4 and BERT. These models are capable of performing a wide range of natural language tasks with a single model architecture.
The term “foundational model” is broader and refers to artificial intelligence models that are not only applied to language processing but can also be trained to perform a variety of tasks in different domains, such as computer vision, audio generation, and more. These models are “foundational” in the sense that they provide a foundation upon which specific applications can be built. Like LLMs, foundational models are typically very large and are trained on huge data sets from multiple modalities and domains. We can say that the foundational models are the base, the initial package from which specific applications can be built.
While LLMs focus on language tasks, foundational models are designed to be even more general, being able to learn from various types of data and apply to a broader range of tasks. LLMs are primarily used in applications that involve text, while foundational models can be used in applications that cross the boundaries between text, image, and other types of data.
- LLMs & SLMs: LLMs (Large Language Models) and SLMs (Small Language Models) have significant differences in terms of size, capabilities, resource requirements and applications.
LLMs have hundreds of millions to billions of parameters. They require large amounts of data and computational resources (processing power, memory and storage) to be trained, so their costs are higher than those of SLMs. LLMs are suitable for applications that require a deep understanding of context and the generation of high-quality text, such as advanced chatbots, virtual assistants and automatic content generation, being able to capture and generate text with a high degree of fluidity and consistency performing complex natural language processing (NLP) tasks such as machine translation, text summarization, and creative content generation.
SLMs have significantly fewer parameters than LLMs, typically in the millions or tens of millions range. They require less data and computational resources, which makes them more accessible for training on less powerful hardware and can be installed in “on premises” mode and work efficiently on devices with lower processing capacity, such as tablets, mobile phones and IoT devices. They are used in applications that require speed and efficiency with less complexity, such as recommendation systems, sentiment analysis on social networks and email classification (SPAM/non-SPAM), being more suitable for solutions that must be deployed on devices with resource limitations.
SLMs are a popular choice for on-premises deployments due to their lower complexity and resource requirements. Organizations in sectors such as finance, healthcare, security, commerce and government are leveraging SLMs for various applications, while ensuring the security and privacy of their data.
- Deterministic Models & Probabilistic Models: Deterministic (stochastic) models and probability-based models are two approaches used to model systems and processes in various disciplines.
Deterministic models do not incorporate any form of randomness. The results are completely determined by the parameters and initial conditions of the model, which makes them highly predictable. Its advantages are its simplicity and clarity in interpretation, and its precise and repeatable results.
Probability-based models incorporate randomness and attempt to capture variability and uncertainty in systems. The results are not unique; instead, they are described in terms of probability distributions. Its advantages are its ability to manage uncertainty and variability, generating a more realistic image of complex systems, allowing the calculation of probabilities and risks associated with different results.
Both types of models have their applications, advantages and limitations. The choice between them depends on the nature of the specific problem being solved, but in general, platforms and tools that have the flexibility to combine deterministic and probabilistic approaches within the same system tend to be more effective, allowing collaboration and complementarity. between both types of models.
- “Closed” & “open” models: this is another false dilemma, since the data confirms that “proprietary” and “open source” models have comparable performances, with a preponderance of one or the other depending on the use cases.
There may be various interactions from the world of “proprietary” systems with the world of free and open-source software, such as contribution to the free software community, integration with open platforms and the use of open-source tools such as open-source libraries to build and improve your capabilities; for example, machine learning frameworks and libraries such as TensorFlow, PyTorch and Scikit-learn, which are open-source projects. This not only improves the functionality of proprietary systems, but it also facilitates integration and adoption by developers who are already familiar with these tools.
We recommend a strategy consisting of building architectures that favor and simplify collaboration between proprietary developments and the open-source community, taking advantage of the best of both worlds, as is the case with some cutting-edge platforms, like IBM Watsonx.
The detailed analysis of previous couples, sometimes confused, often complementary, and always contributing to uncertainty about AI/GenAI, deserves greater depth, which is why I will soon publish another specific article on the topic. In any case, the market offers models, platforms, technologies and tools of all the types mentioned above, with real possibilities of adapting to use cases that will certainly impact (already are doing so) on increased productivity and in the effectiveness of business processes. The real difficulties in deepening the digital journey through the implementation of processes based on AI/GenAI are not the result of these possible confusions: the most difficult thing is to have/build the foundations for said construction, that is, with quality data, duly linked to the organization’s strategy and, with governance processes and the promotion of a data-oriented organizational culture encouraging, stimulating and rewarding innovation and experimentation. Briefly, these are some of the pillars to consider.
- Strategy: The relationship between an organization’s strategy, its data strategy and its ability to transform itself into a data-driven entity, capable of developing successful and effective GenAI initiatives, is fundamental.
The interdependence between the organization’s strategy, its data strategy and its ability to develop GenAI is clear. An organizational strategy that values innovation and technology, together with a well-defined data strategy, lays the foundation for successful GenAI initiatives and digital processes in general.
- Organizational culture: For an organization to follow its “digital journey” becoming a data-driven entity, it is essential adopting several strategic measures linked to its organizational culture, such as creating a Data-driven Oriented Culture, encouraging the adoption of data and promoting its use at all levels of the organization, especially in decision making.
- Digital platforms: Data-oriented digital organizations implement flexible and scalable data infrastructures, capable of handling large volumes of data (big data), both structured and unstructured. They use advanced tools and platforms for data collection, storage, processing and analysis, such as data lakes, data warehouses, lake houses and cloud analytics platforms.
- Governance: Data Governance is required to overcome the main barriers to the digital journey, which are concerns about the lineage or provenance of data, risks related to data security, and restrictions imposed by regulations /compliance. Data Governance is, therefore, a fundamental element of the success of digital transformation and the effective use of AI/GenAI, since it ensures data traceability, quality, security, and accessibility, it complies with regulations, it improves operational efficiency, and it facilitates innovation.
- Innovation and experimentation: A Culture of Innovation and Experimentation must be adopted, promoting the recognition & appreciation of experimentation and continuous learning, ensuring that employees feel stimulated and empowered to try new ideas and learn from failures, counting with the required resources and with the explicit encouragement and support from Senior Management.
Far from trying to compare myself with influential businessmen like Roger McNamee, or with the teams of strategists at Goldman Sachs, I dare to affirm, without fearing being wrong, that AI is not a fraud, no AI bubble is imminent, and those organizations capable of adjusting their strategic compass considering the main pillars of their digital journey, can be sure that they will achieve their objectives improving their productivity, efficiency and effectiveness by properly integrating AI/GenAI and other advanced technologies into their core business processes.
José C. Nordmann
SME in real-time Digital Fraud
Member of the Worldwide Council for a Safer Planet
Member of ACFE (Association of Certified Fraud Examiners)
Member of i2 Group Worldwide Advisory Board