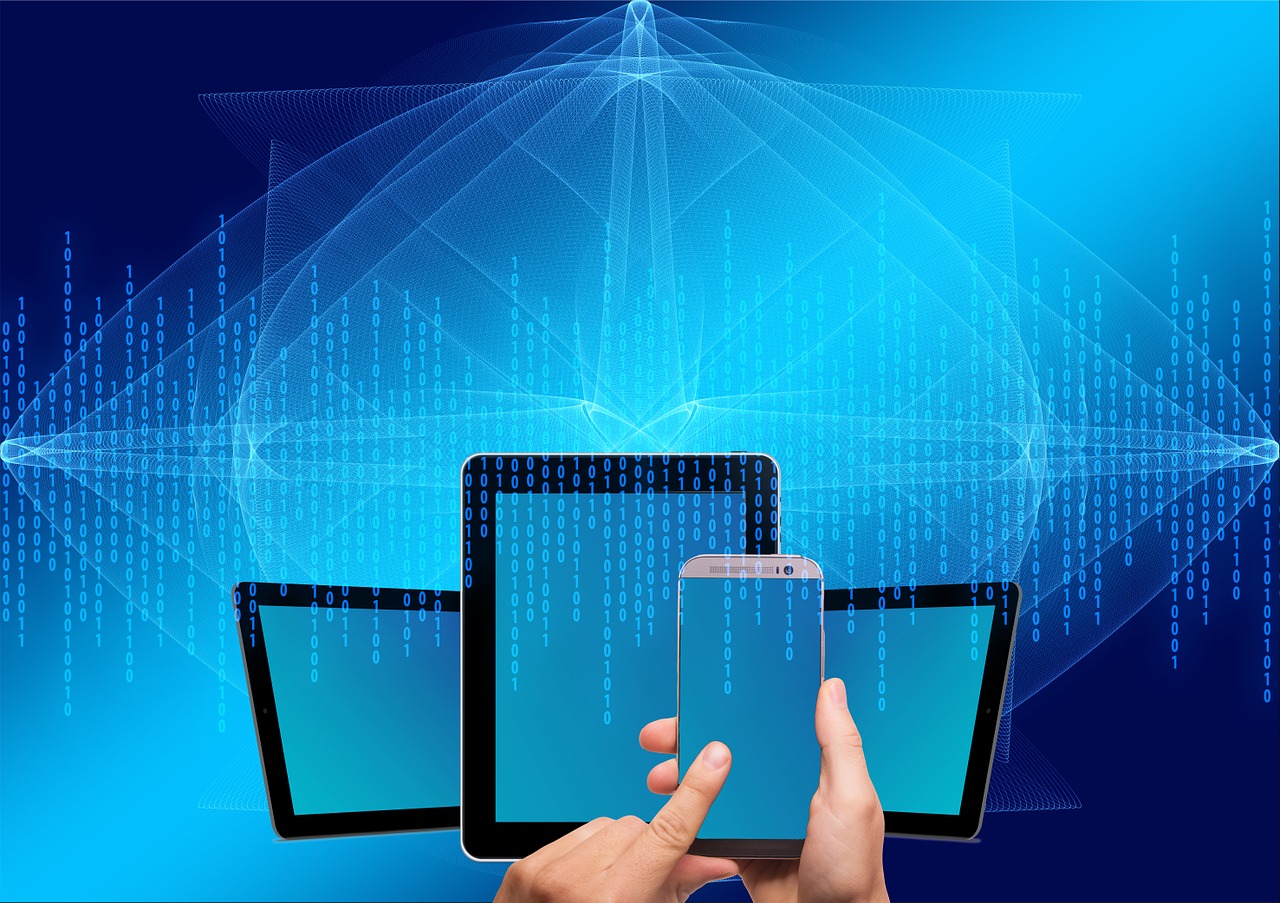
Data Governance in Practice
In the last article we went over some definitions, and problems that can be solved with a Data Governance program. Here we deal with the implementation of two aspects, I consider crucial in data managing: metadata and data quality management, and allso, how they achieve their most when they are harnessed.
Implementing a Data Governance program requires following several steps, before. First, having an organization’s sponsor’s approval, and support to face the cultural change that involves implementing this type of initiatives, and finally, defining the organizational structure that is going to work on the initiatives related to data governance. While it is necessary to assess the starting situation, and which initiatives prioritize, metadata and quality management are usually the first to be implemented.
Metadata Management
Before discussing management, we need to define what we mean by metadata. Technically it is “data on data”, the goal of metadata management is to turn an organization’s data into information and knowledge. There are diverse types of metadata that we can classify into two groups.
Technical metadata. They supply details about source and target systems, database table and field structures, and dependencies of the following kinds of assets:
o host systems, databases, data files and their contents.
o Physical and Logical Models.
o ETL Processes.
o Data quality rules.
o Analytical metadata models.
Business Metadata. Business metadata includes terms, information governance rules, tags, and representatives that provide a context of information that enables effective communication and because everyone in the organization must share the same information and definitions. For instance, the definition of “customer” must be the same throughout an organization. Among its members there may be several concepts: a) those who have already bought at our business, or b) those who are considering making a purchase. It is crucial to agree on what a customer is so that all the organization members mean the same when they all mean the same when they say “customer”
The tool that helps us define terminology through the different areas of the organization is a Data Glossary. This keeps business terms consistency to reduce data asset ambiguous definitions. In addition, it links different types of metadata to give a single and consistent definition, as well as of all the technical assets that participate in its lifecycle.
Data dictionaries have a more technical approach, while data glossaries have a logical perspective to make business terms clear and help each area understand how they relate to the system’s data. A data glossary is more user friendly than a data dictionary, so both business and technical users can resort to it.
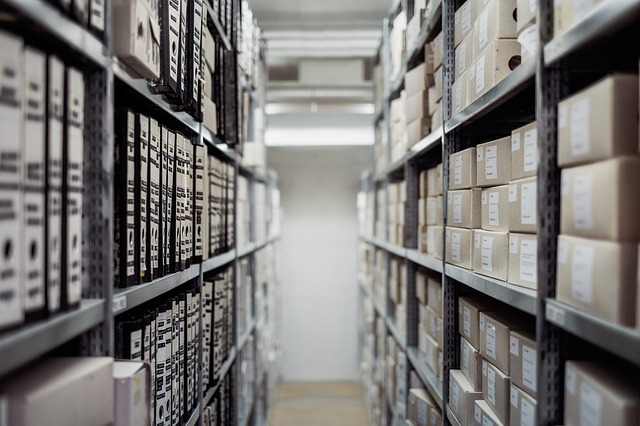
Requirements to create a catalogue:
Plan catalogue structure
Whether a new cataloghe is designed, or taken out of one or more existing glossaries in the company, the process is similar. When developing categories, terms, and their relationships, the terms customized properties must also be planed, for example, their adequate reps and tags. There must also be a definition of principles, scope, policies that will govern our glossary, as well as the metrics to measure compliance with the defined terms.
A catalogue team
A multidisciplinary team is required to be in charge of a catalogue’s governance. The most knowledgeable individuals in the different areas of a company will be spotted to introduce the right content to the catalogue.
Design and development of terms and assets
Creating a glossary includes fulfilling the following tasks:
Corporate terms’ definition.
Definition of customized attributes for terms and categories.
Defining tags for terms, categories and other actives.
Choosing users as representatives.
Relating terms with terms.
Defining actives to be imported to the catalogue.
Loading the catalogue with assets.
Relating terms and assets.
Publishing the glossary
Once the Data Glossary has been implemented it must be published to be used by the organization. Maintenance processes should be arranged, ensuring that the business terms are linked to the technical aspects, and that these have a single meaning for all areas.
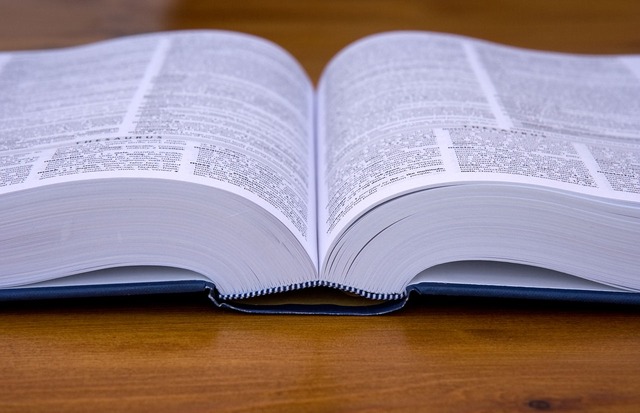
Data Quality Management
Identifying data quality issues, and defining appropriate corrective measures requires whole corporate involvement, not just the effort of an isolated group of people within an organization. It aims to achieve and keep high levels of critical corporate data quality. Data quality management has 3 main axes:
Improvement
Research databases and processes that generate or modify data to correct existing quality issues. This approach presumes there are quality issues to be addressed.
Prevent
Help individuals in several areas build better data checks, better capture processes, better screen layouts, better policies. It is all about permeating quality culture across an organization.
Monitor
Monitor improvements continuously, and preventive actions to measure their effectiveness.
Before we can improve, prevent and monitor these problems must be detected, so 2 approaches are available.
Business approach
This approach looks for poor quality data issues that have a negative impact on your organization’s business processes. This approach applies to complaints, lost customers, reports rejected by central agencies, opportunities lost, wrong decisions, lack of contact data or sale of new products. One disadvantage of this approach is that you need to be very close to the business to detect these issues, which takes effort and time to analyze whether the causes are really due to data problems.
Data approach
This approach looks for data problems. Firstly, a set of rules is defined, which has to be met to have quality data. This requires a good knowledge of data, its definition, values, relationships, etc. The next step is to apply data profiling to confirm or complete the generated metadata. After having checked data quality, business analysts produce evidence of data problems, by applying those rules, This information is key to do research and correct the causes that generate such problem. This approach is easier to implement than the previous approach, as it is less time consuming, less dimensioned in the group that detects problems, and manages to find problems that the other approach would not find (if it does not impact or otherwise the impact of that data is not detected on the business problem. In return, data can often be valid for the rules but not for business processes, which may have a negative impact before the problem is detected.
The business approach with reactive features is the one that will lead us to manage data cleanliness and correction, and then monitor them. Data approach is more proactive, the collaboration between terms definition to build a glossary and define what rules they should comply with, makes profiling and monitoring activities take place first. Therefore, problems will be detected from this phase. A good data quality program should resort to both approaches to capture most part of data problems.
Conclusion
Both metadata management and quality management are key activities within a Data Governance initiative. Their correct management allows corporations to define business terms, how they relate to systems, tables, reports, data models, and the corresponding quality rules determine their level of trust or potential problems to improve, prevent and monitor data quality.
Ing. Gustavo Mesa @gmesahaisburu
Data & Analytics consultant